Table Of Content
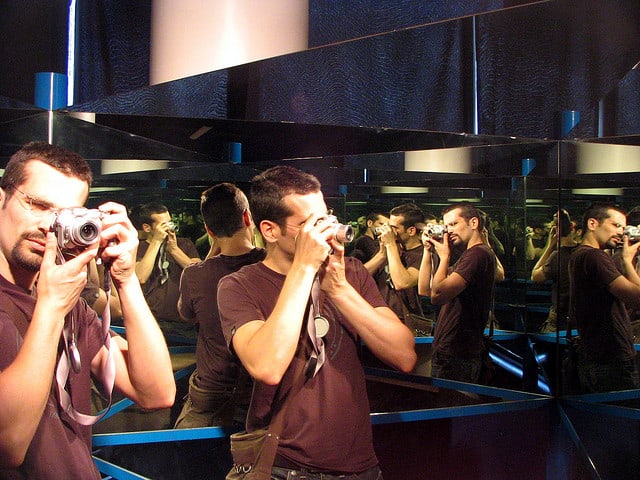
This thought experiment will be our entry point into discussing interactions. A take-home message before we begin is that some independent variables (like shoes and hats) do not interact; however, there are many other independent variables that do. Manipulation checks are usually done at the end of the procedure to be sure that the effect of the manipulation lasted throughout the entire procedure and to avoid calling unnecessary attention to the manipulation. Manipulation checks become especially important when the manipulation of the independent variable turns out to have no effect on the dependent variable. Imagine, for example, that you exposed participants to happy or sad movie music—intending to put them in happy or sad moods—but you found that this had no effect on the number of happy or sad childhood events they recalled. This could be because being in a happy or sad mood has no effect on memories for childhood events.
Clinical research study designs: The essentials
The two conditions should be identical except that the control condition lacks one or more ICs or features that are provided to the active condition. The random assignment of participants to the treatment arms means that the two groups of assigned participants should differ systematically only with regard to exposure to those features that are intentionally withheld from the controls. In smoking cessation research a common RCT design is one in which participants are randomly assigned to either an active pharmacotherapy or to placebo, with both groups also receiving the same counseling intervention. Factorial experiments can be used when there are more than two levels of each factor. However, the number of experimental runs required for three-level (or more) factorial designs will be considerably greater than for their two-level counterparts.
5.1. Correlational Studies With Factorial Designs¶
In the figure, the area selected in black is where the responses will be inputted. For instance, if the purity, yield, and residual amount of catalyst was measured in the DOE study, the values of these for each trial would be entered in the columns. Additional modifications to the design include randomizing and renumbering the design. These are very straightforward modifications which affect the ordering of the trials. For information about the "Fold design" and "Add axial points", consult the "Help" menu. To have a total of 3 trials of each, the user should add 2 replicates in this menu.
Factorial Designs¶
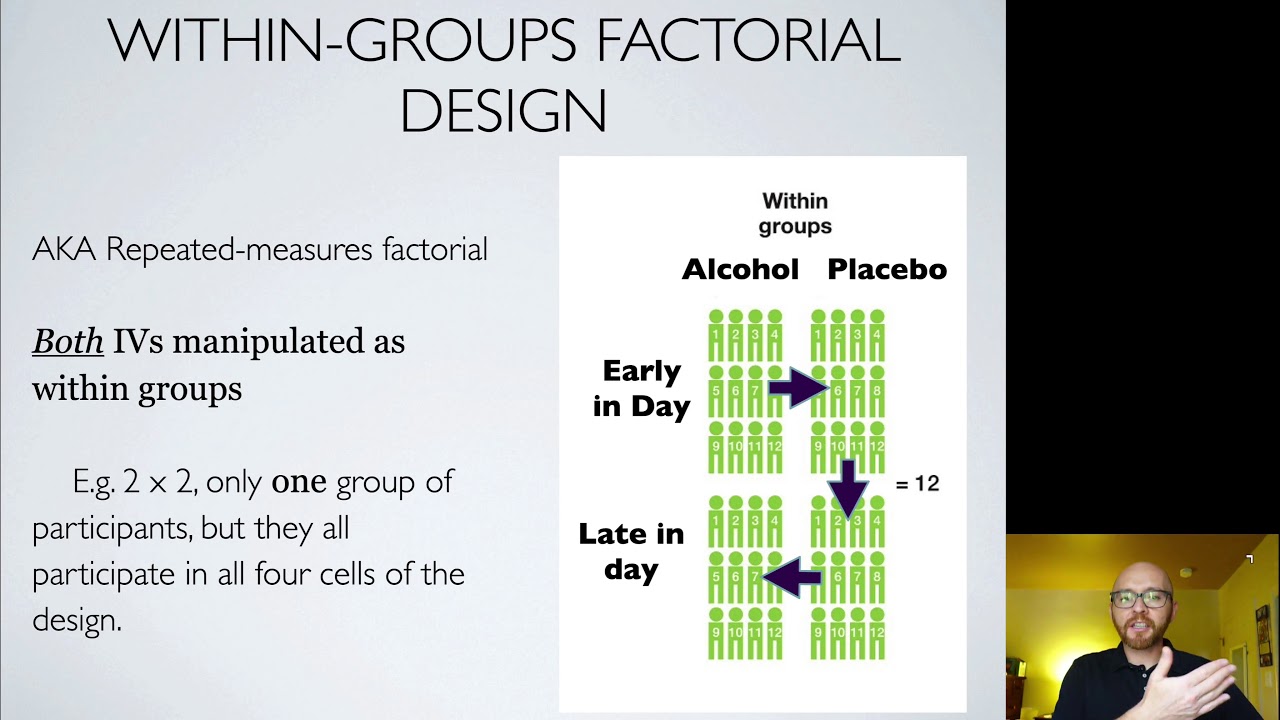
Neither one of these conditions would be a control condition in a strict sense, since each delivers a different form of active treatment. In addition, an RCT might have a control condition, but this might be used in comparisons with many active treatment conditions. For instance, in the comparative treatment design, multiple active treatment conditions are contrasted with a single common control condition (e.g., each of four conditions might receive a different active medication, and each is then compared with a single placebo condition). When researchers study relationships among a large number of conceptually similar variables, they often use a complex statistical technique called factor analysis. In essence, factor analysis organizes the variables into a smaller number of clusters, such that they are strongly correlated within each cluster but weakly correlated between clusters. Each cluster is then interpreted as multiple measures of the same underlying construct.
Optimization of Toluenediamine degradation in synthetic wastewater by a UV/H2O2 process using full factorial design - ScienceDirect.com
Optimization of Toluenediamine degradation in synthetic wastewater by a UV/H2O2 process using full factorial design.
Posted: Thu, 06 Jul 2023 07:35:04 GMT [source]

Also notice that each number in the notation represents one factor, one independent variable. So by looking at how many numbers are in the notation, you can determine how many independent variables there are in the experiment. 2 x 2, 3 x 3, and 2 x 3 designs all have two numbers in the notation and therefore all have two independent variables. The numerical value of each of the numbers represents the number of levels of each independent variable.
IX. Chapter 9: Factorial Designs
Clinical trials are further divided into randomized clinical trial, non‐randomized clinical trial, cross‐over clinical trial and factorial clinical trial. By doing this, psychologists can see if changing the independent variable results in some type of change in the dependent variable. As with any statistical experiment, the experimental runs in a factorial experiment should be randomized to reduce the impact that bias could have on the experimental results. A contrast in cell means is a linear combination of cell means in which the coefficients sum to 0. Contrasts are of interest in themselves, and are the building blocks by which main effects and interactions are defined. The expected response to a given treatment combination is called a cell mean,[12] usually denoted using the Greek letter μ.
The following Yates algorithm table using the data from second two graphs of the main effects section was constructed. Besides the first row in the table, the main total effect value was 10 for factor A and 20 for factor B. However, since the value for B is larger, dosage has a larger effect on percentage of seizures than age. This is what was seen graphically, since the graph with dosage on the horizontal axis has a slope with larger magnitude than the graph with age on the horizontal axis.
V. Chapter 5: Experimental Research
By adding up the coefficient effects with the sub-effects (multiply coefficient with sub-effect), a total factorial effect can be found. This value will determine if the factor has a significant effect on the outcome. For larger numbers, the factor can be considered extremely important and for smaller numbers, the factor can be considered less important. The sign of the number also has a direct correlation to the effect being positive or negative.
Typically, subjects with the disease tend to remember certain events compared to subjects without the disease. Observer bias is a systematic error when the study investigator is influenced by the certain characteristics of the group, that is, an investigator may pay closer attention to the group receiving the treatment versus the group not receiving the treatment. One of the ways to decrease observer bias is to use blinding (discussed in section “Blinding”).
The green bar for no-shoes is slightly smaller than the green bar for shoes. Some questions to ask yourself are 1) can you identify the main effect of wearing shoes in the figure, and 2) can you identify the main effet of wearing hats in the figure. Both of these main effects can be seen in the figure, but they aren’t fully clear. We have usually no knowledge that any one factor will exert its effects independently of all others that can be varied, or that its effects are particularly simply related to variations in these other factors. When there is a large interaction, main effects have little practical meaning as a significant interaction often masks the significance of main effects.
The Big Five personality factors have been identified through factor analyses of people’s scores on a large number of more specific traits. For example, measures of warmth, gregariousness, activity level, and positive emotions tend to be highly correlated with each other and are interpreted as representing the construct of extraversion. As a final example, researchers Peter Rentfrow and Samuel Gosling asked more than 1,700 university students to rate how much they liked 14 different popular genres of music [RG03]. They then submitted these 14 variables to a factor analysis, which identified four distinct factors. These ideas can be confusing if you think that the word “independent” refers to the relationship between independent variables.
In this example, the employment sector and gender of the graduates are the independent variables, and the starting salaries are the dependent variables. So, for example, a 4×3 factorial design would involve two independent variables with four levels for one IV and three levels for the other IV. A full factorial design, also known as fully crossed design, refers to an experimental design that consists of two or more factors, with each factor having multiple discrete possible values or “levels”. A factorial design is often used by scientists wishing to understand the effect of two or more independent variables upon a single dependent variable. The advantage of multiple regression is that it can show whether an independent variable makes a contribution to a dependent variable over and above the contributions made by other independent variables. As a hypothetical example, imagine that a researcher wants to know how the independent variables of income and health relate to the dependent variable of happiness.
A null outcome situation is when the outcome of your experiment is the same regardless of how the levels within your experiment were combined. From the example above, a null outcome would exist if you received the same percentage of seizures occurring in patients with varying dose and age. The graphs below illustrate no change in the percentage of seizures for all factors, so you can conclude that the chance of suffering from a seizure is not affected by the dosage of the drug or the age of the patient. The main disadvantage is the difficulty of experimenting with more than two factors, or many levels. A factorial design has to be planned meticulously, as an error in one of the levels, or in the general operationalization, will jeopardize a great amount of work.
This is tricky because income and health are themselves related to each other. Thus if people with greater incomes tend to be happier, then perhaps this is only because they tend to be healthier. Likewise, if people who are healthier tend to be happier, perhaps this is only because they tend to make more money. But a multiple regression analysis including both income and happiness as independent variables would show whether each one makes a contribution to happiness when the other is taken into account.
The bottom panel of Figure 5.3 shows the results of a 4 x 2 design in which one of the variables is quantitative. This variable, psychotherapy length, is represented along the x-axis, and the other variable (psychotherapy type) is represented by differently formatted lines. This is a line graph rather than a bar graph because the variable on the x-axis is quantitative with a small number of distinct levels. Line graphs are also appropriate when representing measurements made over a time interval (also referred to as time series information) on the x-axis.
No comments:
Post a Comment